We discussed correlation vs causation and confounding factors in our
previous post.
Confounding is a distortion of the association between an exposure and an outcome that occurs when participants of the study differ with respect to other factors that influence the outcome. This bias is not introduced by the investigator, laboratory or by the subjects, and it can be adjusted for in the analysis afterwards.
MEBO is a complex condition and while some of the cases seem simpler, non-linear analysis approaches are better equipped to handle the task. And this creates new challenges regarding the existence of confounding factors.
What are there potential confounding factors that could distort the true relationship between microbes identified in this study and the risk of MEBO symptom flareups?
Age is one of the most studied confounding variables.
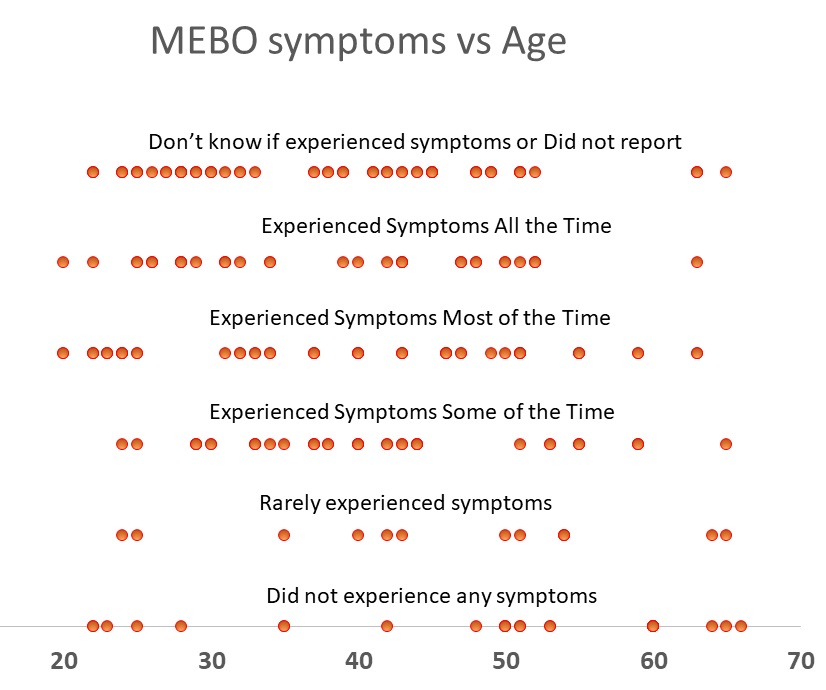
It is known that aging changes the smell of a person - mostly because aging-related altered forms of enzymes produce 2-nonenal from diet-dependent sweat-secreted omega-7 fatty acids.
2-nonenal is detectable only in people over 40. Mean age in our study and control populations is about 40 and it is slightly different for males and females (see baseline demographic table) but the difference is not significant
enough to
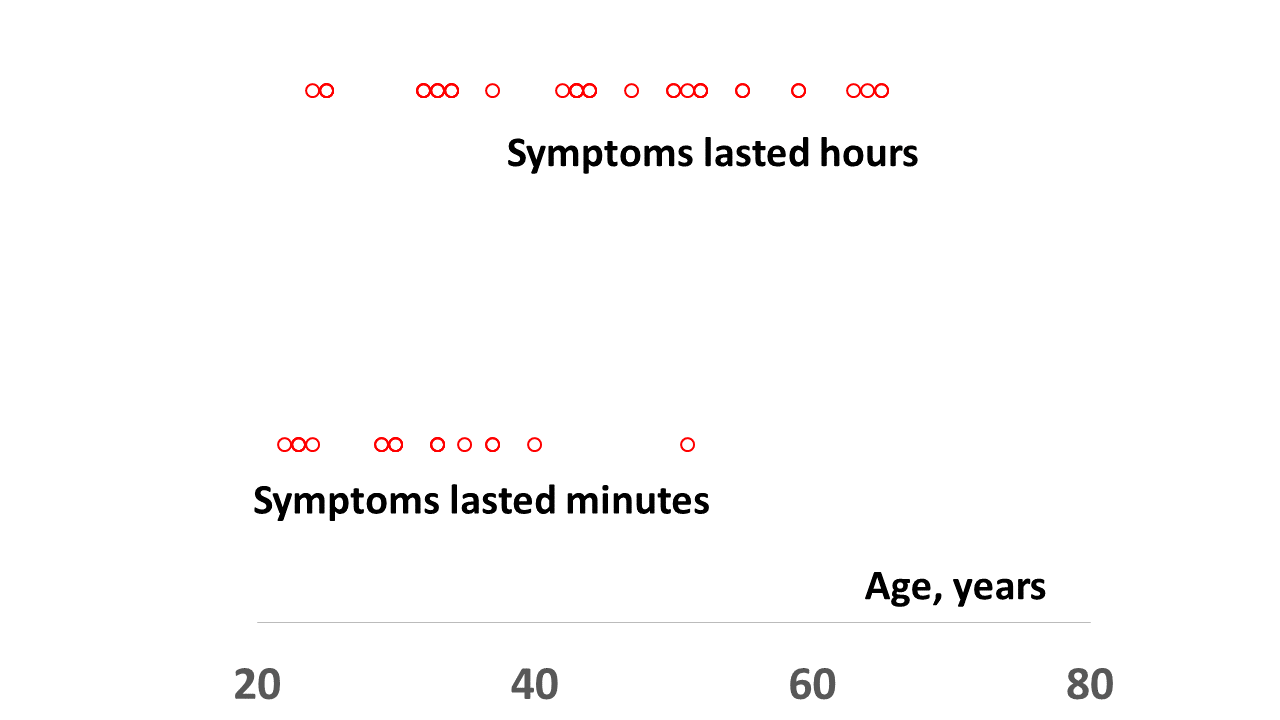
make it a confounding variable. Is there anything else that is statistically different?